class: center, middle, inverse, title-slide # Networks Worksheet ### Mathew Kiang ### 2/17/2017 --- class: center, middle # Networks ## Guys, networks are awesome. .footnote[.red[*]Like all labs, I'm just helping you walk through the code — you gotta figure out the rest of the worksheet on your own.] --- class: center, middle # Download the code.red[*] ## [https://git.io/vDSzh](https://git.io/vDSzh) # Also download the data ## [https://git.io/vDSgT](https://git.io/vDSgT) --- class: center, middle # Let's first walk through the function we gave you again. ## You really don't need to know this. This is entirely for your own personal edification. --- ## Code review — networkPractical() ```r networkPractical <- function(network, attackRate, acquireImmunity, runTime) { #we will encode susceptible individuals as 0, infected as 1, immune as 2 numberOfPeople <- network.size(network) #size of the population #start out with everyone susceptible statusList <- array(0,dim=c(numberOfPeople,1)) timeSeries <- NULL keepStatus <- NULL #INFECT THE FIRST PERSON! statusList[1] <- 1 ``` `networkPractical` just takes a few arguments. -- - `network` is a network object that you give it -- - `attackRate` is the probability of infection between an I and an S (conditional on them being connected by an edge) -- - `acquireImmunity` is the probability of gaining immunity after infection -- - `runTime` is just how long you want to run the simulation. --- ## Code review — networkPractical() ```r networkPractical <- function(network, attackRate, acquireImmunity, runTime) { #we will encode susceptible individuals as 0, infected as 1, immune as 2 * numberOfPeople <- network.size(network) #size of the population #start out with everyone susceptible statusList <- array(0,dim=c(numberOfPeople,1)) timeSeries <- NULL keepStatus <- NULL #INFECT THE FIRST PERSON! statusList[1] <- 1 ``` First, just find the number of people in your population (in this case, the network) --- ## Code review — networkPractical() ```r networkPractical <- function(network, attackRate, acquireImmunity, runTime) { #we will encode susceptible individuals as 0, infected as 1, immune as 2 numberOfPeople <- network.size(network) #size of the population }} #start out with everyone susceptible * statusList <- array(0,dim=c(numberOfPeople,1)) timeSeries <- NULL keepStatus <- NULL #INFECT THE FIRST PERSON! statusList[1] <- 1 ``` Then we are going to make a list that contains the status of each node. We will it up with 0 because everybody is susceptile. (Here, we are just declaring the number of `dim`ensions to be 1 colum with as many rows are there are people). --- ## Code review — networkPractical() ```r networkPractical <- function(network, attackRate, acquireImmunity, runTime) { #we will encode susceptible individuals as 0, infected as 1, immune as 2 numberOfPeople <- network.size(network) #size of the population }} #start out with everyone susceptible statusList <- array(0,dim=c(numberOfPeople,1)) }} * timeSeries <- NULL * keepStatus <- NULL #INFECT THE FIRST PERSON! statusList[1] <- 1 ``` We just make two other objects (empty) that we will use later in our loops. (Recall from previous labs that if you make an object inside of a loop, it gets overwritten every time you run it.) --- ## Code review — networkPractical() ```r networkPractical <- function(network, attackRate, acquireImmunity, runTime) { #we will encode susceptible individuals as 0, infected as 1, immune as 2 numberOfPeople <- network.size(network) #size of the population }} #start out with everyone susceptible statusList <- array(0,dim=c(numberOfPeople,1)) }} timeSeries <- NULL keepStatus <- NULL #INFECT THE FIRST PERSON! * statusList[1] <- 1 ``` Infect somebody. In this case, we are infecting the first person, but we could also just infect somebody randomly. We use `1` to signify infected (recall `0` is for suspcetibles). --- ## Code review — networkPractical() ```r *for(time in 1:runTime) { * print(time) whoIsInfected <- which(statusList==1) whoIsImmune <- which(statusList==2) if(length(whoIsInfected)==numberOfPeople | length(whoIsImmune)==numberOfPeople | length(whoIsInfected)==0) {} ``` Now we get to the good stuff. We are going to run a for() loop for as many timesteps as specified in `runTime`. We print it just so we have some idea of whether or not the loop is going. --- ## Code review — networkPractical() ```r for(time in 1:runTime) { print(time) * whoIsInfected <- which(statusList==1) * whoIsImmune <- which(statusList==2) if(length(whoIsInfected)==numberOfPeople | length(whoIsImmune)==numberOfPeople | length(whoIsInfected)==0) {} ``` Recall from previous labs that `which()` just returns the **index** (row-number in this case) for which the condition is true. So we are asking `whoIsInfected`? And we get back a list that tells us which row has an infected status `1`. (Also recall that each row represents a node or person so in this case you can think of it as an ID as well.) -- Obviously, the first time you run this, nobody will be Immunte and only one (the one we assign) will be infected, but it changes each time we go through the `for` loop. --- ## Code review — networkPractical() ```r for(time in 1:runTime) { print(time) whoIsInfected <- which(statusList==1) whoIsImmune <- which(statusList==2) * if(length(whoIsInfected)==numberOfPeople | * length(whoIsImmune)==numberOfPeople | * length(whoIsInfected)==0) {} ``` This is just creating a stop condition. The pipe `|` means `or`. If (1) everybody is infected or (2) everybody is immunte or (3) nobody is infected, then run what is inside the bracket. Since nothing is in the bracket, it will break out of the loop. --- ## Code review — networkPractical() ```r else { for(i in 1:length(whoIsInfected)){ #only look at people who are infected #see who is connected to infected node contacts <- get.neighborhood(network, whoIsInfected[i]) #see which contacts are susceptible to infection suscContacts <- which(statusList[contacts]==0) ``` That's all boring though. This section starts what to do if you have both infected and susceptibles. Go through everybody who is infected. Get all their contacts (`get.neighborhood`) and then find the ones that are susceptible. --- ## Code review — networkPractical() ```r if(length(suscContacts)>0) { #see which infectious contacts lead to infection successfulInfect <- which(runif(length(suscContacts))<attackRate) if(length(successfulInfect)>0){ #INFECT SUSCEPTIBLES! statusList[contacts[suscContacts[successfulInfect]]] <- 1 } ``` If there are any that are susceptible (that is, if `length(suscContacts) > 0`), try to infect them. Here, we use a random uniform (`runif`) to draw a number between `\([0, 1]\)`. If that number is less than our attack rate, we infect them. Otherwise, we do nothing. The second half then says, if anybody was infected (`successfulInfect`), change their status to 1 to indicate they were infected. --- ## Code review — networkPractical() ```r if(length(whoIsInfected)>0){ #see who becomes immune becomeImmune <- which(runif(length(whoIsInfected)) < acquireImmunity) statusList[whoIsInfected[becomeImmune]] <- 2 } ``` Now let's look at everybody who is infected, and again, randomly assign them to immune using the `runif`. This is the same as above, just using the random uniform number to assign immunity instead of infection. --- ## Code review — networkPractical() ```r S <- length(which(statusList==0)) I <- length(which(statusList==1)) R <- length(which(statusList==2)) timeSeries <- rbind(timeSeries,c(S,I,R)) #keep track of people keepStatus <- cbind(keepStatus,statusList) ``` Now, every time we do this loop (remember we are doing this `runTimes` many times), record the number of susceptible, infected, and recovered. Then bind that to the `timeSeries` object we made above. Similarly, keep track of all the statuses for every timestep in the `keepStatus` object. --- class: center, middle, inverse # Question 1: Install the network package ## You got this one. --- ## But in case you don't: ```r ## Install it install.packages("network") ## Load it library(network) ``` --- class: center, middle, inverse # Question 2: Import the networks ## You got this one too --- ## Remember, the GUI is your friend. ```r library(readr) blah <- read_delim("./data/network1.txt", delim = " ", col_names = FALSE, trim_ws = TRUE) mat1 <- read_delim("./data/mat1-1.txt", delim = " ", col_names = FALSE, trim_ws = TRUE) mat3 <- read_delim("./data/mat1-3.txt", delim = "\t", col_names = FALSE, trim_ws = TRUE) mat4 <- read_delim("./data/mat3.txt", delim = "\t", col_names = FALSE, trim_ws = TRUE) matBA <- read_delim("./data/ba_net.txt", delim = " ", col_names = FALSE, trim_ws = TRUE) ``` **NOTE**: Some of these are delimited with a tab (`"\t"`) and some are whitespace delimited (`" "`). Use the GUI so you can see a live preview and modify accodingly. Also don't forget there are no headers. --- ## Convert them to networks ```r net1 <- as.network(blah, directed = FALSE) net3 <- as.network(mat3, directed = FALSE) net4 <- as.network(mat4, directed = FALSE) ## Note: These have nodes labeled 0. We need to add 1 ## so that the zeros are not there. mat1 <- mat1 + 1 matBA <- matBA + 1 net2 <- as.network(mat1, directed = FALSE) netBA <- as.network(matBA, directed = FALSE) ``` --- ## Plot the networks — net1 ```r plot.network(net1, vertex.cex = 1.5) ``` 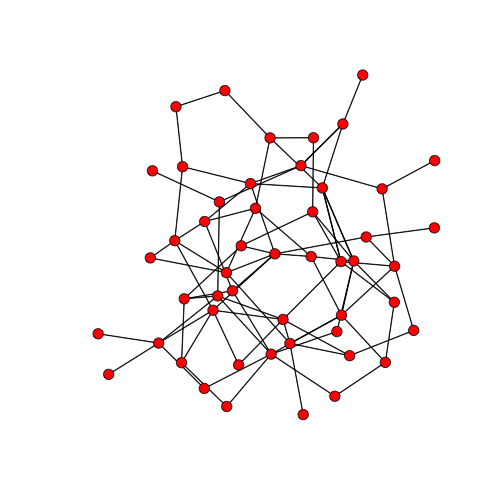<!-- --> --- ## Plot the networks — net2 ```r plot.network(net2, vertex.cex = 1.5) ``` 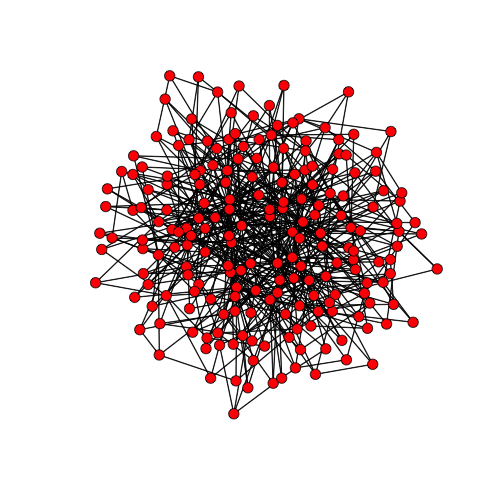<!-- --> --- ## Plot the networks — net3 ```r plot.network(net3, vertex.cex = 1.5) ``` 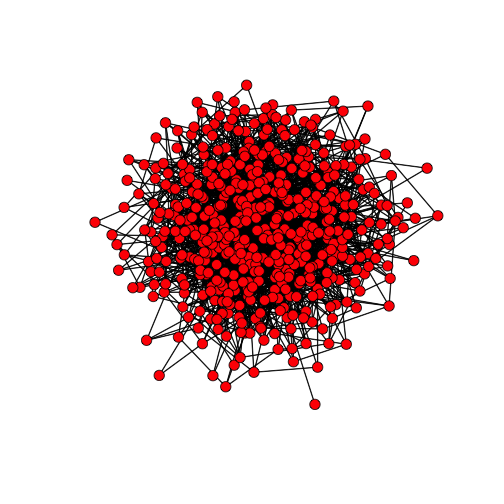<!-- --> --- ## Plot the networks — net4 ```r plot.network(net4, vertex.cex = 1.5) ``` 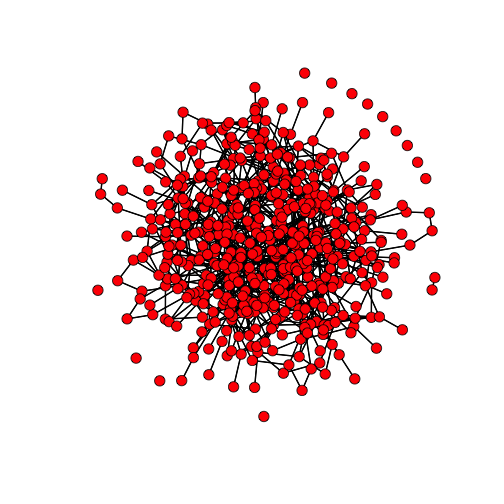<!-- --> --- ## Plot the networks — netBA ```r plot.network(netBA, vertex.cex = 1.5) ``` 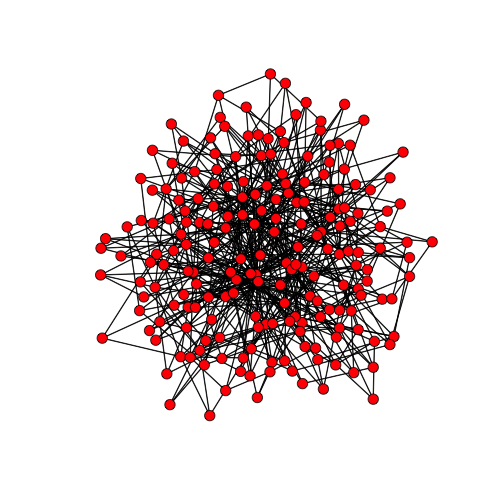<!-- --> --- class: center, middle # What's the point? -- ### Hopefully, it's clear by looking at the plots of the networks what we want to learn here. How does the structure of the network affect disease dynamics? What about networks with isolates and more than one connected component? What about dense vs sparse networks? --- class: center, middle, inverse # Question 3. Degree distributions --- # What's this code do? ```r deg <- NULL for(i in 1:network.size(net1)) { deg <- rbind(deg, length(get.neighborhood(net1, i))) } ``` -- - For every node in the network... -- - Go through and get the number of neighbors (`length(get.neighborhood())`). -- - And append it to an object called `deg`. -- - That's it. Just get the number of neighbors for every node in a network. --- # Degree distribution — net1 ```r deg_net1 <- NULL for(i in 1:network.size(net1)) { deg_net1 <- rbind(deg_net1, length(get.neighborhood(net1, i))) } hist(deg_net1) ``` 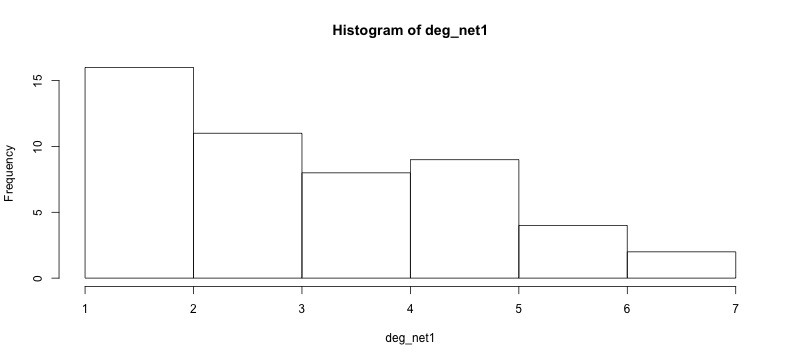<!-- --> --- # Degree distribution — net2 ```r deg_net2 <- NULL for(i in 1:network.size(net2)) { deg_net2 <- rbind(deg_net2, length(get.neighborhood(net2, i))) } hist(deg_net2) ``` 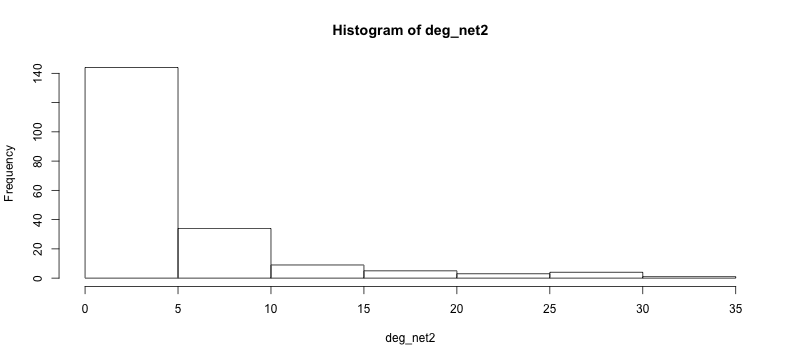<!-- --> --- # Degree distribution — net3 ```r deg_net3 <- NULL for(i in 1:network.size(net3)) { deg_net3 <- rbind(deg_net3, length(get.neighborhood(net3, i))) } hist(deg_net3) ``` 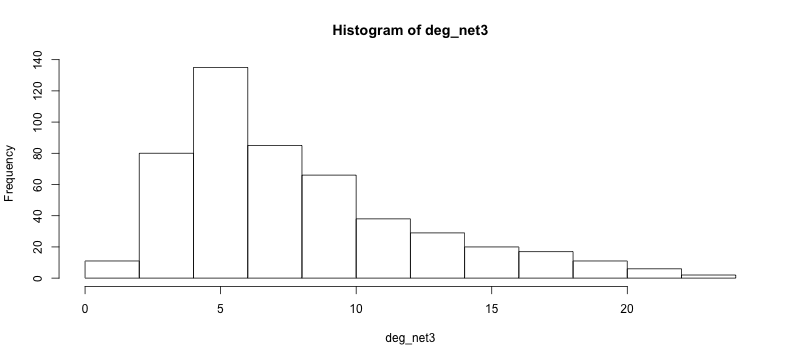<!-- --> --- # Degree distribution — net4 ```r deg_net4 <- NULL for(i in 1:network.size(net4)) { deg_net4 <- rbind(deg_net4, length(get.neighborhood(net4, i))) } hist(deg_net4) ``` 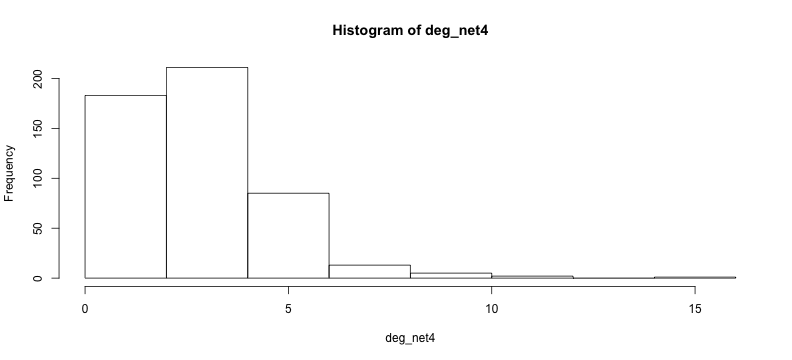<!-- --> --- # Degree distribution — netBA ```r deg_netBA <- NULL for(i in 1:network.size(netBA)) { deg_netBA <- rbind(deg_netBA, length(get.neighborhood(netBA, i))) } hist(deg_netBA) ``` 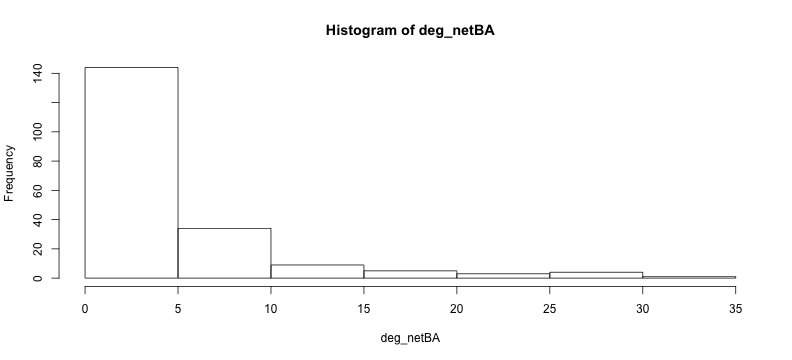<!-- --> --- class: center, middle, inverse # Question 4. Simulating on a network --- # Simulate on net2 ```r sim_net2a <- networkPractical(network = net2, attackRate = .2, acquireImmunity = .2, runTime = 50) ``` ```r matplot(sim_net2a$timeSeries, type = "l", lty = 1) ``` 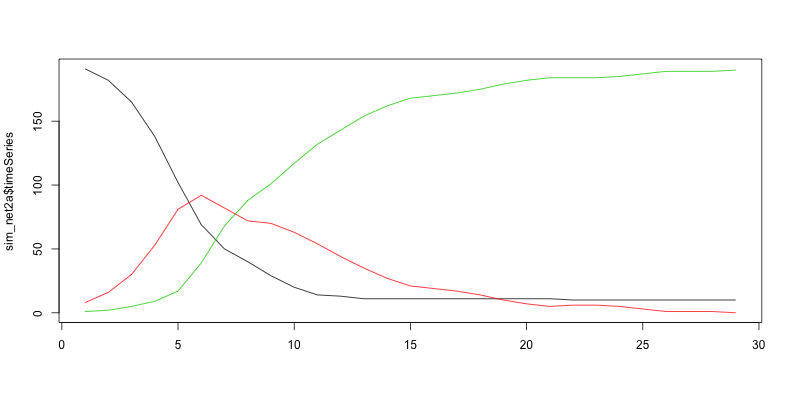<!-- --> --- # Simulate on net2 ```r matplot(sim_net2a$timeSeries, type = "l", lty = 1) ``` 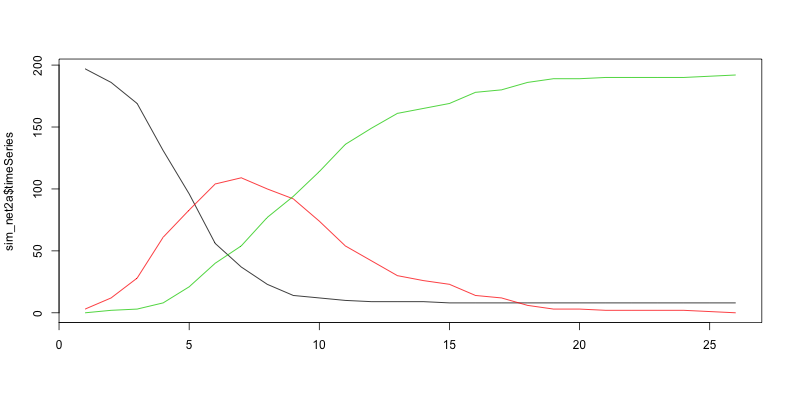<!-- --> **NOTE**: Every run results in a different plot. Why? --- class: center, middle, inverse # Question 5. Plot the network with node status --- # Plot with status ```r plot.network(net2, vertex.col = sim_net2a$status[, 10] + 2, vertex.cex = 1.5) legend("topright", fill = c(2, 3, 4), legend = c("S", "I", "R")) ``` 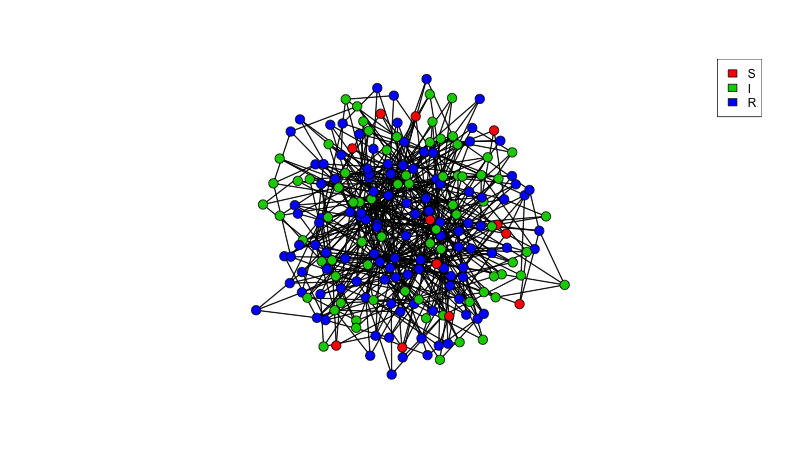<!-- --> --- class: center, middle, inverse # Questions 6 - 9 ## Just use previous slides with different parameters. Change timesteps. Plot two infectious curves on the same plot. Play with it a bit. We give you the code so you can play with the numbers and see what happens. Don't worry about the code too much. --- class: center, middle, inverse # That's it. ## Thanks